Artificial intelligence (AI) can be understood essentially as the use of devices which have the capacity to mimic human cognitive processes, in turn, capable of learning and solving complex problems [1]. The AI nomenclature was publicly introduced at the Dartmouth conference in 1956, since then, there have been significant advances, especially in the application of AI models in images, contributions which corroborated the resurgence of the theme in mid-2012, being denominated as a pillar for the “fourth industrial revolution” [2]. Within the scenario of health services, it was not different, due to the potential of AI models being able to reduce the time until diagnosis in several chronic-degenerative diseases [3-5]. Despite notable and expressive advances, AI is understood by health professionals as a single device, a fact that is misinterpreted, AI involves a set of terms which are hierarchically divided into: machine learning (ML), deep learning (DL) and convolutional neural networks (CNNs) (Figure 1) [6].
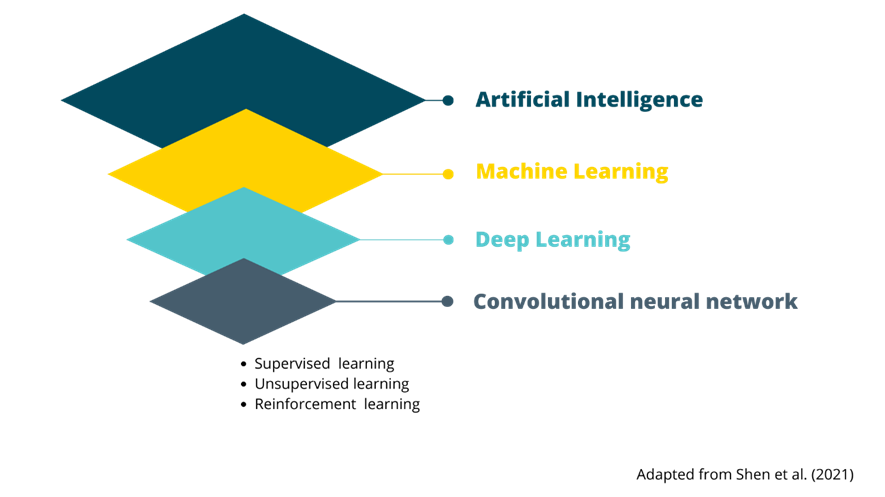
ML is generically understood as the mapping of inputs and outputs in a data record, particularly, ML is largely dedicated to the implementation of algorithms without the need for prior programming, a process called train test, consisting of training, testing and validation. However, considering the type of task, ML can be divided into three categories: supervised, unsupervised and reinforcement learning [6].
Regarding DL, it is based on multiple layers of artificial neural network processing, having the ability to automatically learn information representations and accumulate experiences based on raw data, resulting in a decrease in the use of specialized human resources. The various layers of DL provided a significant advance in several areas, due to the high processing capacity of large databases, as well as the development of learning algorithms [6].
CNNs are a subcategory of artificial neural networks, however, the input type is mainly considered images, being effective and efficient particularly in the medical imaging scenario. CNN layers are divided into four types:
1): convolutional layer: filter-based learning;
2): pooling layer: the function of this layer is similar to a small-world network, that is, it has the principles of reducing the sample of resource maps, also controlling overfitting;
3): fully connected layer: acts to predict classification labels;
4): non-linearity layer: considers specific conditions in images for activation or not of neurons, resulting in a wide capacity to learn hierarchical functions and abstract characteristics in the context of medical images [6].
About the application of AI models in medical images, current advances show that the implementation of these models in different organs and systems (Figure 2), are effective in differentiating lesions, tumors or malefic nodules and benefits by allocating the need for the use of high-cost therapies, contributing to reducing the excessive use of material and human resources and the time until diagnosis in several chronic-degenerative diseases [3-6].
Figure 2. Some of the AI applications in the medical imaging landscape.
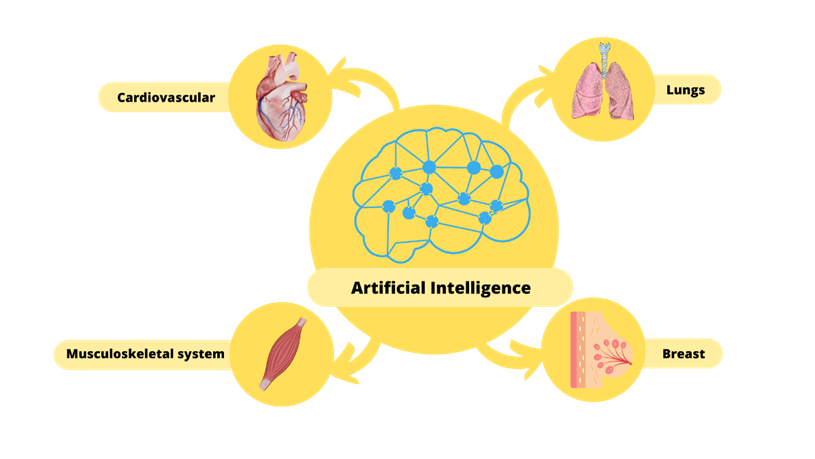
Citations
[1] S.L. Goldenberg, G. Nir, S.E. Salcudean, A new era: artificial intelligence and machine learning in prostate cancer, Nat. Rev. Urol. 16 (7) (2019) 391–403.
[2] V. Mnih, K. Kavukcuoglu, D. Silver, A.A. Rusu, J. Veness, M.G. Bellemare, A. Graves, M. Riedmiller, A.K. Fidjeland, G. Ostrovski, S. Petersen, C. Beattie, A. Sadik, I. Antonoglou, H. King, D. Kumaran, D. Wierstra, S. Legg, D. Hassabis, Human-level control through deep reinforcement learning, Nature 518 (7540) (2015) 529–533.
[3] J.H. Chen, S.M. Asch, Machine learning and prediction in medicine – beyond the peak of inflated expectations, N. Engl. J. Med. 376 (26) (2017) 2507–2509.
[4] E.J. Topol, High-performance medicine: the convergence of human and artificial intelligence, Nat. Med. 25 (1) (2019) 44–56.
[5] K.H. Yu, A.L. Beam, I.S. Kohane, Artificial intelligence in healthcare, Nat. Biomed. Eng. 2 (10) (2018) 719–731.
[6] Shen YT, Chen L, Yue WW, Xu HX. Artificial intelligence in ultrasound. Eur J Radiol. 2021 Jun;139:109717.